Microscopic imaging often faces resolution limitations, hindering detailed structural analysis crucial for scientific fields like biology and materials science. While techniques such as SRGAN, ESRGAN, and SwinIR improve resolution, they often fail to preserve structural integrity, impacting tasks like identifying cellular structures and examining material defects. In response, our research aims to develop a strategy to integrate structural information into super-resolution techniques. By using the intricacies of conditional generative adversarial networks (cGANs) and optimizing them through a structure-informed convex loss function, we aim to enhance the quality of super-resolution images.
Oshadi Perera
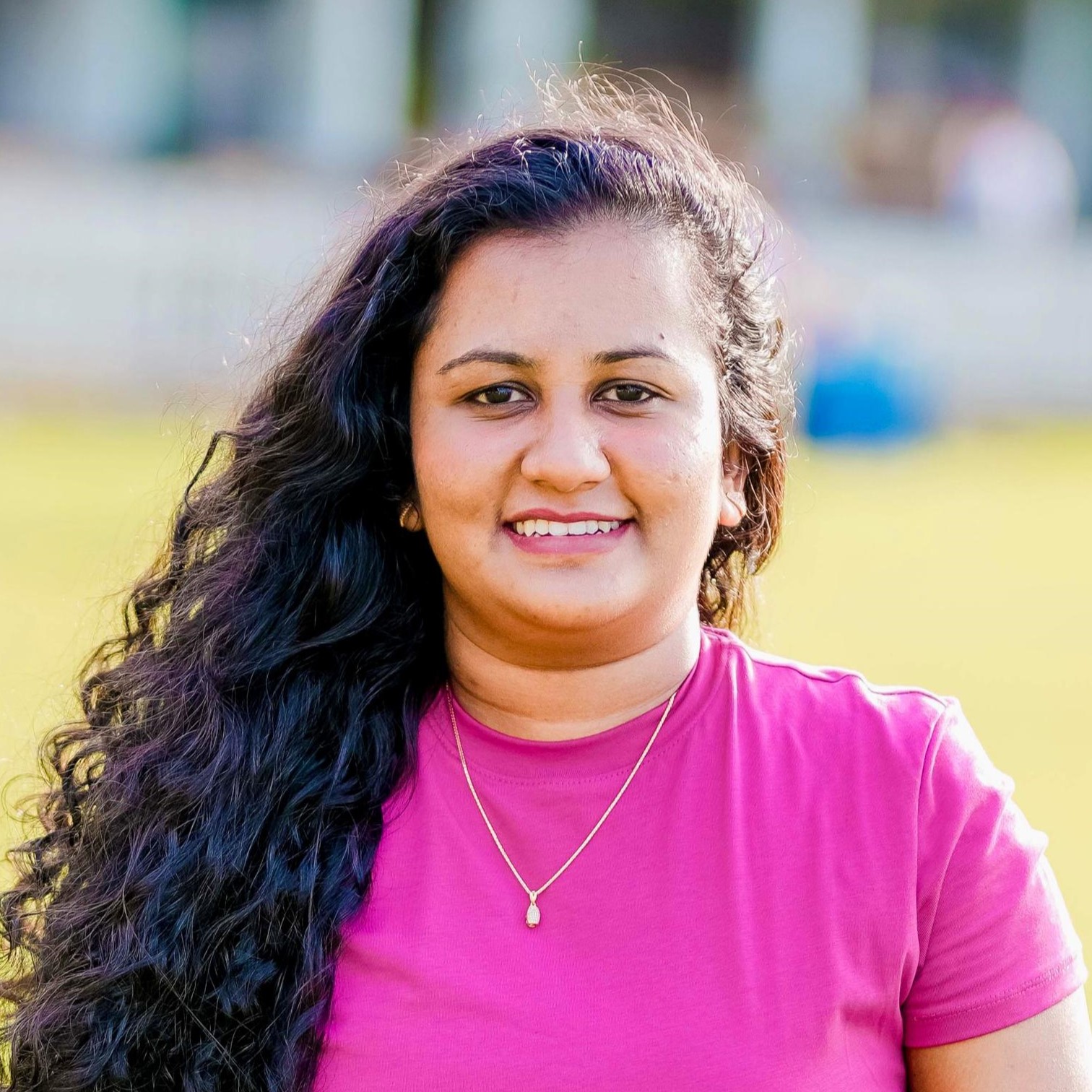
Enthusiastic Data Science Engineering undergraduate with a strong academic background and fervent passion for Machine Learning, Data Science, and Artificial Intelligence. Fueled by curiosity and an unyielding drive to learn, empowered by a diverse skill set and a relentless spirit, I aspire to create a lasting impact through intelligent solutions.