In panel data forecasting, no single model consistently performs well across diverse panel data characteristics, such as varying entity counts, time steps, and data distributions. Traditional models often struggle to adapt to non-stationarity, diverse patterns, and complex entity interactions within panel data. This limitation underscores the need for robust deep-learning solutions that can handle these challenges and improve forecasting in critical fields like economics, environment, transport, and healthcare. Therefore, developing generalizable, robust deep learning architectures that can adapt to varying panel data characteristics is crucial to improve forecasting accuracy in these critical fields. We are going to build a robust panel time series algorithm using deep learning that works well in any kind of dataset characteristics.
Shabthana Johnson
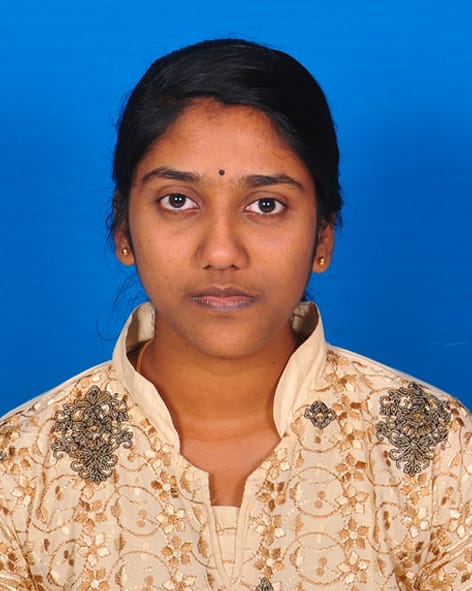
I am a fourth-year undergraduate student at the University of Moratuwa, specializing in Computer Science and Engineering. My academic and professional journey is driven by a strong passion for Data Science, with a focus on applying Machine Learning and Deep Learning techniques to areas such as time series analysis and Natural Language Processing (NLP). Currently, I am working on developing a robust panel time series algorithm using Deep Neural Networks to advance time series forecasting. I am committed to continuous learning and actively expand my skills through self-study and practical experience with emerging technologies.